Storing tables efficiently with Pandas
- 2019-04-03
- ⚙️ DE
- Python Benchmark Performance
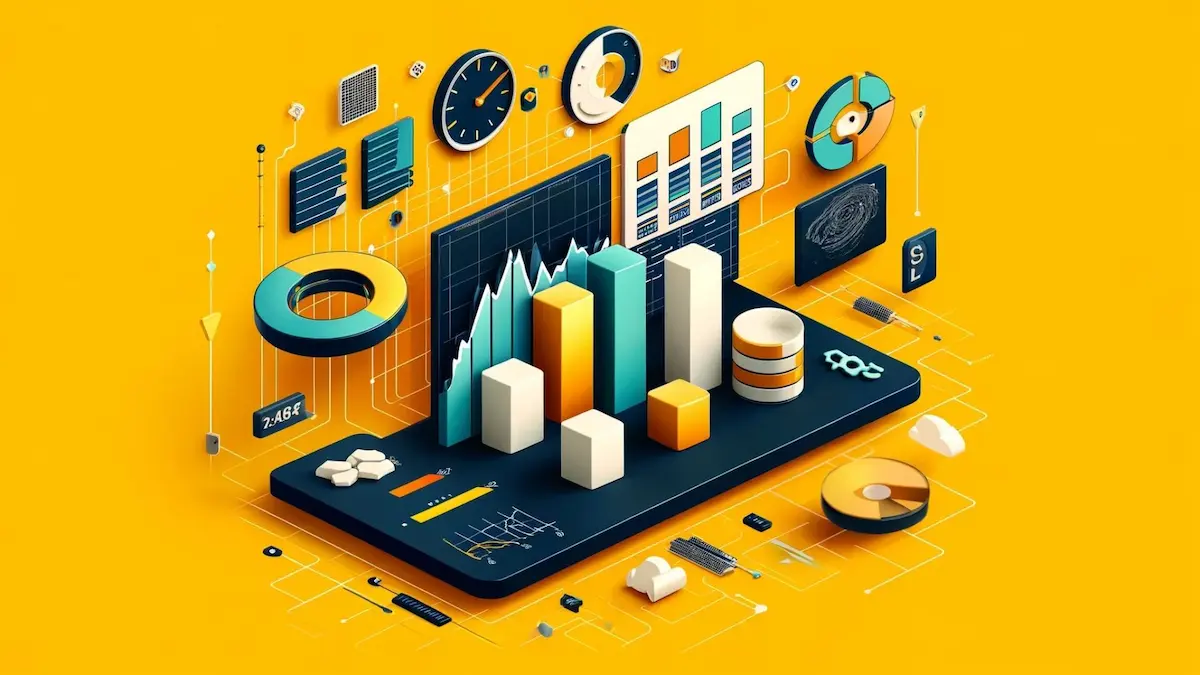
0. Intro
When working with pandas people usually need to store one or more tables. There are a lot of different formats to do that. In this post I am going to compare the performance between them.
1. How test will be done
To do the tests I downloaded 3 datasets of different sizes:
I downloaded them from kaggle, one of the best places to find datasets.
The formats that I will test are:
- csv
- feather
- msgpack
- parquet
- pickle
- xlsx
2. Test 1: Simple test
First of all I will check how they perform without changing any parameters. I will do 100 iterations with the small dataset and 10 with the medium one.
2.1. Size small and iterations 100 with all extensions
First of all the average reading/writing times for each format.
It seems that xlsx
is a slow option
2.2. Size medium and iterations 10 with all extensions
With the medium dataframe the results are very similar.
xlsx
is a slow solution. I would only recommend it for small dataframes.
Regarding the file size both xlsx
and parquet
outperform the rest. If you look the pandas documentation you will see that all extensions except xlsx
and feather
allow different types of compression. Also parquet
uses a more agresive compression by default. We need to test the formats and the different compressions.
3. Test 2: Include compressions
This time each extensions will be tested using all posible compressions. The results are:
format | compression | read [s] | write [s] | size [MB] |
---|---|---|---|---|
csv | None | 3.05 | 5.90 | 236.10 |
bz2 | 10.52 | 24.44 | 47.58 | |
gzip | 4.26 | 49.02 | 65.80 | |
infer | 3.09 | 6.00 | 236.10 | |
xz | 9.92 | 288.62 | 43.99 | |
zip | 4.05 | 21.26 | 66.44 | |
feather | feather | 0.61 | 1.00 | 213.38 |
msgpack | None | 0.49 | 1.32 | 210.09 |
blosc | 0.48 | 1.37 | 202.88 | |
zlib | 0.51 | 2.05 | 202.90 | |
parquet | None | 0.77 | 1.41 | 209.58 |
brotli | 0.99 | 27.04 | 50.82 | |
gzip | 1.00 | 18.28 | 56.75 | |
snappy | 0.81 | 1.87 | 96.37 | |
pickle | None | 0.47 | 1.34 | 207.54 |
bz2 | 7.78 | 18.51 | 40.93 | |
gzip | 1.49 | 40.68 | 54.85 | |
infer | 0.48 | 1.37 | 207.54 | |
xz | 6.26 | 254.32 | 38.05 | |
zip | 2.07 | 17.86 | 55.43 |
By plotting both reading and writing time is easier to se that writing times for xz
compression are really slow.
It is not clear which formats and compressions work best. Let’s plot reading/writing time vs file size.
csv underperforms compared to the other formats
4. Test 3: Using the big table
For this test I will only use the best formats from the previous test. Those are:
- feather
- msgpack
- parquet
- pickle
format | compression | read [min] | write [min] | size [GB] |
---|---|---|---|---|
feather | feather | - | - | 4.24 |
msgpack | None | 5.86 | 6.32 | 7.21 |
blosc | 5.45 | 5.01 | 6.50 | |
zlib | 5.49 | 5.55 | 6.50 | |
parquet | None | 1.15 | 2.69 | 5.96 |
brotli | 0.92 | 11.22 | 1.56 | |
gzip | 0.97 | 8.98 | 2.00 | |
snappy | 0.89 | 2.78 | 3.30 | |
pickle | None | 1.38 | 7.47 | 6.25 |
bz2 | 5.15 | 17.47 | 1.24 | |
gzip | 1.88 | 20.76 | 1.81 | |
infer | 1.46 | 5.80 | 6.25 | |
xz | 3.46 | 97.62 | 0.97 |
feather did not work since it has a restriction of 2 GB per column and it was exceeded.
With a file of this size it is clear that parquet is the best option. For compression it depends if your priority is file size or Input/Output time. For fast writing/reading use parquet without compression, for minimum file size blosc
and zlib
is a solution that is between the other two.
parquet
is the best for big tables.
5. Conlusions
Excel
is only useful for small files when you are planning to open with an Excel program.csv
performs poorlypickle
,msgpack
andparquet
are good optionsfeather
is another option when the table is not bigparquet
is a good option in general and the best one for big files.parquet without compression
is the fastest solution for big files andparquet with blosc
the best at compressing big files.